RespLLM: Unifying Audio and Text with Multimodal LLMs for Generalized Respiratory Health Prediction
Can large language models (LLMs) transform respiratory disease screening through automatic consultation and auscultation?
Imagine an AI assistant capable of "listening" as well as "reading", incorporating sounds and textual information into insights that can assist in diagnosing respiratory conditions earlier and more accurately. RespLLM, provides an innovative application of the OPERA model that demonstrates how LLMs can potentially be transformed into your personal respiratory health assistants. This approach leverages the strengths of LLMs to analyze complex respiratory sounds alongside patient-reported symptoms, medical histories and so on, providing enhanced insights and flexibility that traditional models cannot offer.
Respiratory diseases remain a significant public health challenge, characterized by high incidence and mortality rates. Early screening is essential for improving patient outcomes, yet traditional methods often fall short in addressing the complexities involved in accurately assessing respiratory health. Recent advances in machine learning, particularly through large language models (LLMs), offer an opportunity to improve clinical consultations and auscultation, leading to better early detection and management of respiratory conditions.
A major hurdle in respiratory health assessment is the complexity and heterogeneity of the data involved. Clinical evaluations typically rely on a range of information, including demographic details, medical history, symptom descriptions, and auscultation of respiratory sounds. This complexity and heterogeneity in data can hinder the performance of existing machine learning models, which often rely on limited training data, simple fusion techniques, and task-specific approaches. Such limitations can affect the models' ability to generalize across different scenarios and datasets.
To address these challenges, we introduce RespLLM, a novel multimodal large language model framework designed to integrate text and audio information for predicting respiratory health. RespLLM harnesses the extensive knowledge embedded in pretrained LLMs, effectively enabling the integration of audio and text data through attention mechanisms. This approach allows for a more nuanced understanding of the relationships between various types of data, enhancing the model's predictive capabilities.
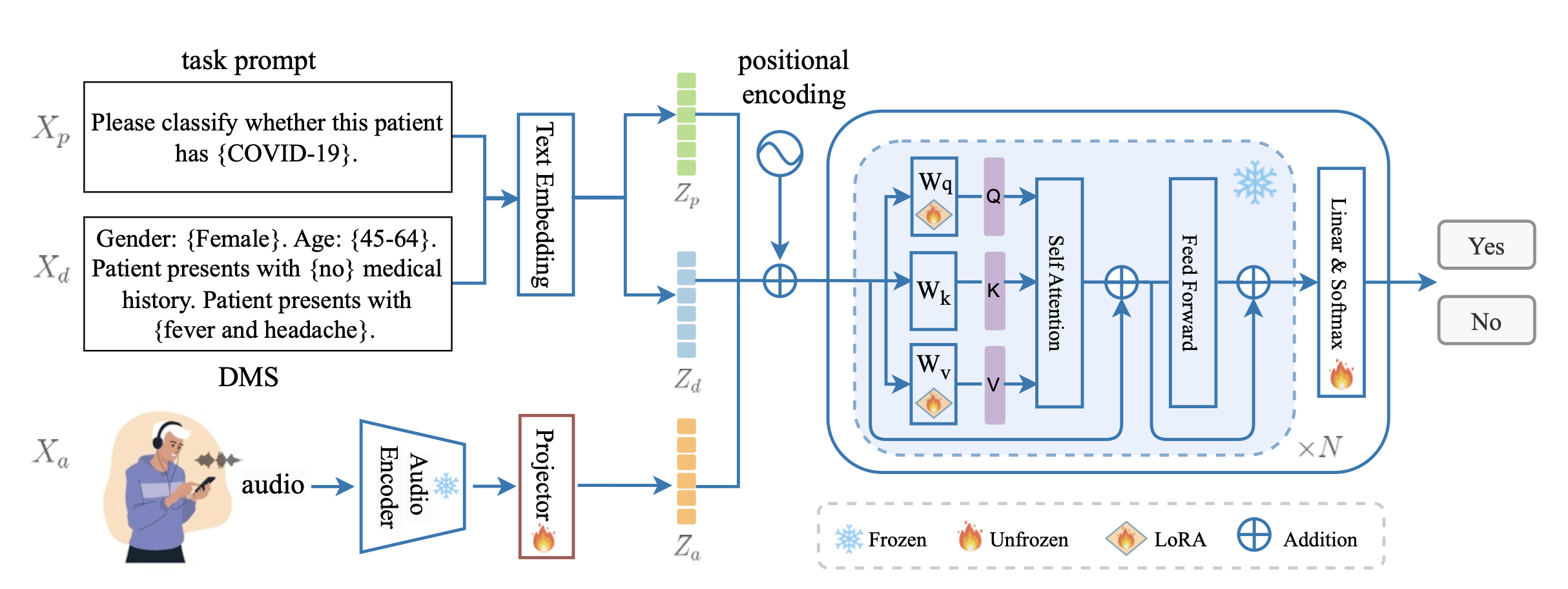
Incorporating instruction tuning, RespLLM benefits from multiple data sources covering diverse tasks. This makes the model more versatile and generalizable, capable of adapting to a wide array of respiratory health scenarios.
Experiments conducted on five real-world datasets demonstrate the effectiveness of RespLLM. The results show that RespLLM outperforms leading baselines by an average of 4.6% on trained tasks and 7.9% on unseen datasets. This substantial improvement underscores the model's ability to generalize beyond its training data, allowing for rapid adaptability in clinical settings.
The implications of RespLLM extend beyond mere performance metrics. By paving the way for multimodal models that can perceive, listen to, and understand heterogeneous data, we are moving toward a future where respiratory health screening can be scaled and improved. This innovative approach not only enhances diagnostic accuracy but also holds the potential to transform clinical workflows, ultimately benefiting patients and healthcare providers alike.
Reference
-
Zhang Y, Xia T, Han J, Wu Y, Rizos G, Liu Y, Mosuily M, Chauhan J, Mascolo C. Towards open respiratory acoustic foundation models: Pretraining and benchmarking. In Thirty-eighth Conference on Neural Information Processing Systems Datasets and Benchmarks Track, 2024.
-
Zhang Y, Xia T, Saeed A, Mascolo C. RespLLM: Unifying Audio and Text with Multimodal LLMs for Generalized Respiratory Health Prediction. In Machine Learning for Health, 2024, PMLR.