OPERA Blog
Can AI leveraging respiratory sounds reveal our health status? The answer is yes, but the real question is: how?
While acoustic AI is advancing rapidly in areas like speech recognition and virtual assistants, progress in health-related acoustic AI has been slower. A major obstacle is the scarcity of health-related audio data and pathological labels. Our system, OPERA, addresses this challenge by curating large-scale unlabelled respiratory audio datasets to pretrain audio encoders that can be adapted for various health tasks with limited labeled data. More importantly, OPERA is an open system, promoting accessibility and transparency in the safety-critical healthcare domain.
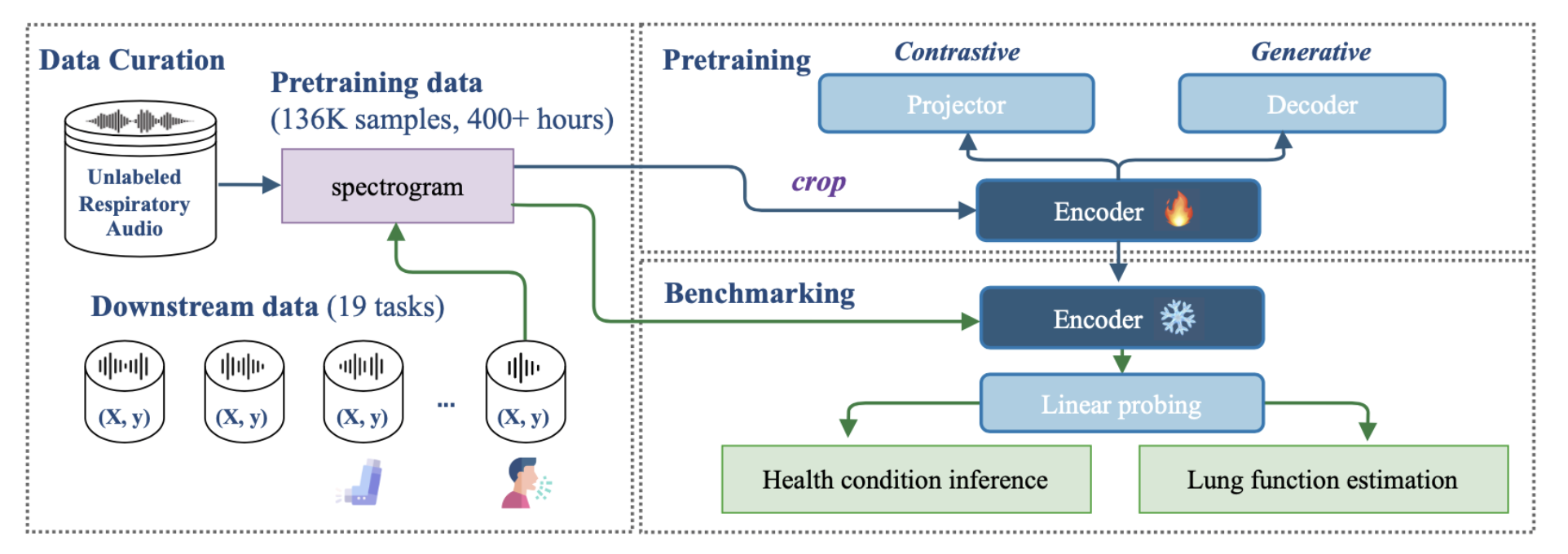
OPERA curated a unique large-scale, unlabeled respiratory audio database (~136K samples, 404 hours) from five diverse sources. The audio covers breathing, coughing, and lung sounds, with all data publicly available (or under controlled access). This dataset is significantly larger than those used for training existing open acoustic models, providing a crucial resource for advancing health-related acoustic AI.
The large-scale data enables us to pretrain foundational audio encoders. Since no labels are available, we deployed and compared two common self-supervised learning (SSL) strategies: contrastive learning, which captures similar representations for similar respiratory audio segments, and generative pretraining, which reconstructs the whole spectrum from parts of the audio. By relying solely on the audio itself, we ensure the generalizability of the pretrained encoders. These encoders can then be used as feature extractors for downstream health applications.
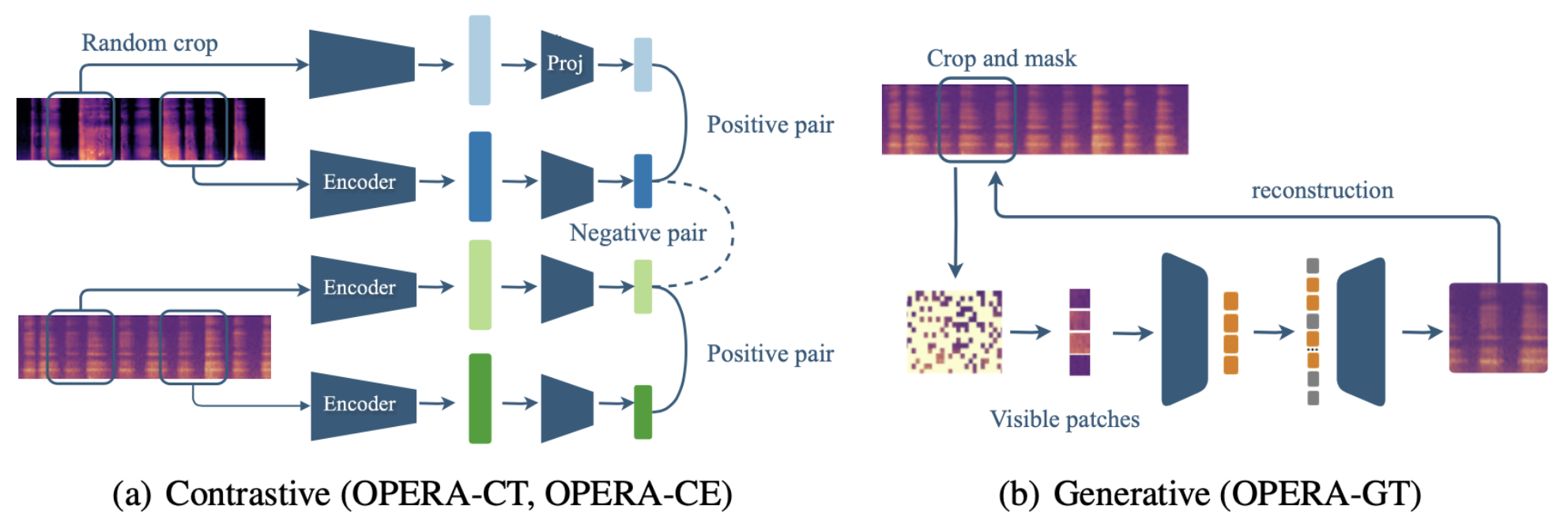
In addition to pretraining, OPERA offers 10 labeled datasets (6 not covered in pretraining) organized into 19 respiratory health tasks, including 12 focused on health condition inference and 7 on lung function estimation. This allows for a fair, comprehensive, and reproducible evaluation. Using linear probing, we directly assess the efficiency of the extracted representations. On this benchmark, our pretrained models outperform existing acoustic models on 16 out of 19 tasks and demonstrate strong generalization to unseen datasets and new respiratory audio types.
SSL and foundation models are gaining momentum in machine learning for health because they reduce the labeling burden while maintaining generality and performance. OPERA marks a critical first step toward creating comprehensive and reproducible audio foundation models for health. Future research can build upon OPERA as an experimental resource, and healthcare applications can benefit from our foundation models as powerful feature extractors. This work expands the potential of machine learning, which now not only sees (through vision) and reads (through language) but also listens to our health (through audio).
Reference
Check the paper introducing OPERA datasets, pretrained models and the benchmark.
- Zhang Y, Xia T, Han J, Wu Y, Rizos G, Liu Y, Mosuily M, Chauhan J, Mascolo C. Towards open respiratory acoustic foundation models: Pretraining and benchmarking. In Thirty-eighth Conference on Neural Information Processing Systems Datasets and Benchmarks Track, 2024.